❓ #DidYouKnow that #explainableai can help us better understand #machinelearning (ML) models?
🧠 Modern ML-models are getting more complex while being successfully applied to an increasing variety of applications and domains like health, cars, and manufacturing. At the same time, more and more cases are becoming known in which #AI models worked differently than intended, for example, by making racist or sexist decisions [1].
⚖️ Amongst others, this leads to regulatory discussions regarding the explainability of ML-models, like the GDPR’s “right to explanation” [2]. Likewise, the German BaFin has published principles for using algorithms in decision-making processes in finance, including instructions on handling the choice between model complexity and interpretability [3].
💡 An easy way to ensure explainability is the application of inherently #interpretable models like small #decisiontrees or simple linear #regression models. However, those models often have less predictive performance compared to other models like deep #neuralnetworks. An alternative approach uses more powerful “black-box” models and specific explainable AI (#XAI) techniques.
🕵️ One of the most common approaches to shed light on an ML-model’s decision is to uncover which parts of the input had the most influence on the output. This principle applies to various types of inputs like images, words, or tabular data. For example, for skin cancer detection in images, a #heatmap can indicate which image regions were particularly important for detecting skin cancer [4].
👩💻 There are many different algorithms for determining which input parts influence a model’s prediction. Some approaches can be applied only to certain model types, e.g., tree-based models or #neuralnetworks [5], while others can uncover influential inputs for any model [6].
Reference links:
Contributing Editor: Nina Schaaf, Women in AI & Robotics volunteer, team #Stuttgart.
#explainableai#interpretableml#didyouknow#womeninai#womeninrobotics#generationequality#ml#artificialintelligence#algorithms
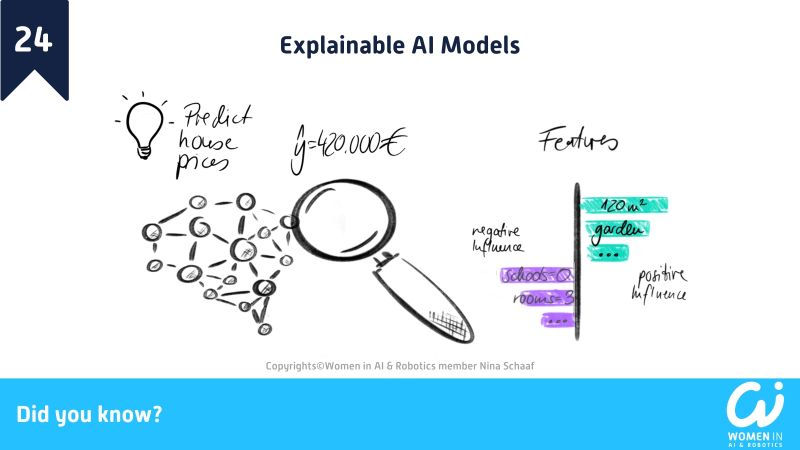
Comments